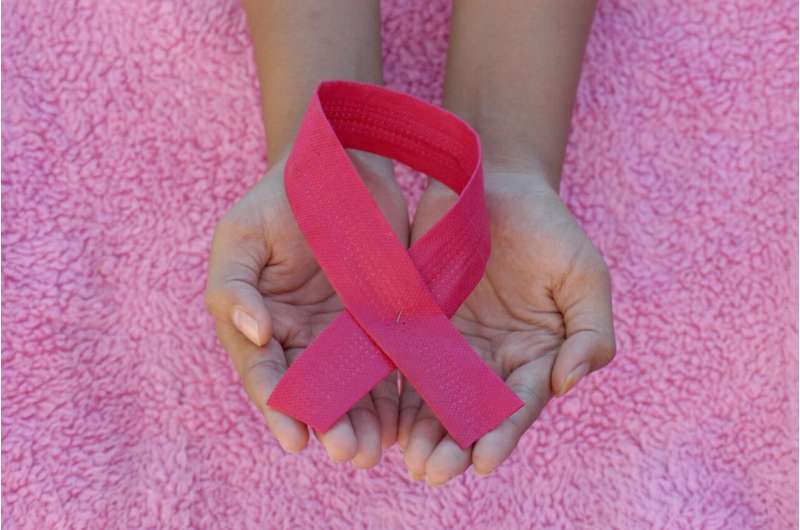
A new AI (artificial intelligence) tool may make it possible to spare breast cancer patients unnecessary chemotherapy treatments by using a more precise method of predicting their outcomes, reports a new Northwestern Medicine study.
AI evaluations of patient tissues were better at predicting the future course of a patient's disease than evaluations performed by expert pathologists.
The AI tool was able to identify breast cancer patients who are currently classified as high or intermediate risk but who become long-term survivors. That means the duration or intensity of their chemotherapy could be reduced. This is important since chemotherapy is associated with unpleasant and harmful side effects such as nausea, or more rarely, damage to the heart.
Currently pathologist evaluate cancerous cells in a patient's tissue to determine treatment. But patterns of non-cancerous cells are very important in predicting outcomes, the study showed.
This is the first study to use AI for comprehensive evaluation of both the cancerous and non-cancerous elements of invasive breast cancer.
"Our study demonstrates the importance of non-cancer components in determining a patient's outcome," said corresponding study author Lee Cooper, associate professor of pathology at Northwestern University Feinberg School of Medicine. "The importance of these elements was known from biological studies, but this knowledge has not been effectively translated to clinical use."
The study was published Nov. 27 in Nature Medicine.
In 2023, about 300,000 U.S. women will receive a diagnosis of invasive breast cancer. About one in eight U.S. women will receive a breast cancer diagnosis in their lifetime.
During diagnosis, a pathologist reviews the cancerous tissue to determine how abnormal the tissue appears. This process, known as grading, focuses on the appearance of cancer cells and has remained largely unchanged for decades. The grade, determined by the pathologist, is used to help determine what treatment a patient will receive.
Many studies of breast cancer biology have shown that the non-cancerous cells, including cells from the immune system and cells that provide form and structure for the tissue, can play an important role in sustaining or inhibiting cancer growth.
Cooper and colleagues built an AI model to evaluate breast cancer tissue from digital images that measures the appearance of both cancerous and non-cancerous cells, as well as interactions between them.
"These patterns are challenging for a pathologist to evaluate as they can be difficult for the human eye to categorize reliably," said Cooper, also a member of the Robert H. Lurie Comprehensive Cancer Center of Northwestern University. "The AI model measures these patterns and presents information to the pathologist in a way that makes the AI decision-making process clear to the pathologist."
The AI system analyzes 26 different properties of a patient's breast tissue to generate an overall prognostic score. The system also generates individual scores for the cancer, immune and stromal cells to explain the overall score to the pathologist. For example, in some patients, a favorable prognosis score may be due to properties of their immune cells, where for others it may be due to properties of their cancer cells. This information could be used by a patient's care team in creating an individualized treatment plan.
Adoption of the new model could provide patients diagnosed with breast cancers with a more accurate estimate of the risk associated with their disease, empowering them to make informed decisions about their clinical care, Cooper said.
Additionally, this model may help in assessing therapeutic response, allowing treatment to be escalated or de-escalated depending on how the microscopic appearance of the tissue changes over time. For example, the tool may be able to recognize the effectiveness of a patient's immune system in targeting the cancer during chemotherapy, which could be used to reduce the duration or intensity of chemotherapy.
"We also hope that this model could reduce disparities for patients who are diagnosed in community settings," Cooper said. "These patients may not have access to a pathologist who specializes in breast cancer, and our AI model could help a generalist pathologist when evaluating breast cancers."
How the study worked
The study was conducted in collaboration with the American Cancer Society (ACS) which created a unique dataset of breast cancer patients through their Cancer Prevention Studies. This dataset has representation of patients from over 423 U.S. counties, many who received a diagnosis or care at community medical centers. This is important because most studies typically use data from large academic medical centers which represent only a portion of the U.S. population.
In this collaboration, Northwestern developed the AI software while scientists at the ACS and National Cancer Institute provided expertise on breast cancer epidemiology and clinical outcomes.
To train the AI model, scientists required hundreds of thousands of human-generated annotations of cells and tissue structures within digital images of patient tissues. To achieve this, they created an international network of medical students and pathologists across several continents. These volunteers provided this data through a website over the course of several years to make it possible for the AI model to reliably interpret images of breast cancer tissue.
Next the scientists will evaluate this model prospectively to validate it for clinical use. This coincides with the transition to using digital images for diagnosis at Northwestern Medicine, which will happen over the next three years.
The scientists also are working to develop models for more specific types of breast cancers like triple-negative or HER2-positive. Invasive breast cancer encompasses several different categories, and the important tissue patterns may vary across these categories.
"This will improve our ability to predict outcomes and will provide further insights into the biology of breast cancers," Cooper said.
Other Northwestern authors include Mohamed Amgad Tageldin, Kalliopi Siziopikou and Jeffery Goldstein.
More information: A population-level digital historic biomarker for enhanced prognosis of invasive breast cancer, Nature Medicine (2023). DOI: 10.1038/s41591-023-02643-7
Citation: AI may spare breast cancer patients unnecessary treatments (2023, November 27) retrieved 27 November 2023 from https://ift.tt/w4DQ8q1
This document is subject to copyright. Apart from any fair dealing for the purpose of private study or research, no part may be reproduced without the written permission. The content is provided for information purposes only.
"breast" - Google News
November 27, 2023 at 11:00PM
https://ift.tt/RWa45bx
AI may spare breast cancer patients unnecessary treatments - Medical Xpress
"breast" - Google News
https://ift.tt/zk1wdZc
https://ift.tt/j1GBedx
Bagikan Berita Ini
0 Response to "AI may spare breast cancer patients unnecessary treatments - Medical Xpress"
Post a Comment