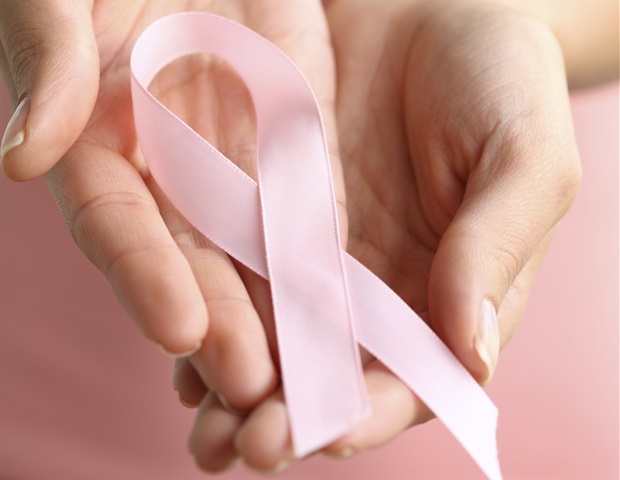
A recently published article from University of Eastern Finland and Kuopio University Hospital reports new innovative use of machine learning to help understand the interplay of genetic and other breast cancer risk factors.
New discoveries in recent years have identified several risk factors contributing to breast cancer risk. Approaches capable of merging genetic (SNPs) and non-genetic risk factors could identify individuals with a high risk of developing cancer and enable the development of risk-adapted screening programs for better cancer prevention. This could potentially improve the overall performance of BC screening and lead to an efficient allocation of clinical resources. The results were published in peer-reviewed Scientific Reports.
A new model created for analyzing interaction of breast cancer risk factors utilizing machine learning
A major challenge in breast cancer risk prediction is to develop a model that incorporates all known and newly found risk factors while considering the interactions among them. The machine learning model developed at the Institute of Clinical Medicine, Pathology and Forensic Medicine at the University of Eastern Finland enables identifying combinations of interacting genetic variants and demographic risk factors of breast cancer, which can efficiently predict the breast cancer risk.
The machine learning approach is based on a gradient tree boosting method followed by an adaptive iterative search algorithm. The best identified features, i.e., interacting SNPs + demographic risk factors, are used to predict the breast cancer risk for an unknown individual. The analysis has been done on the Kuopio Breast Cancer Project (KBCP) data.
Identified gene interaction map include ESR1 and FGFR1 genes, that are linked to estrogen receptor-positive Breast Cancer subtype
Interestingly, the gene interaction map of the SNPs, that were found to interact with the demographic risk factors, points to the prominent estrogen-related-linked network (ESR1 network). The gene interaction map additionally points to the FGFR2 gene, which is one of the most important genetic susceptibility loci in non-hereditary breast cancer.
"Since cancer is a multi-factorial disease caused by lifestyle, genetic, and environmental factors, individual analysis of the sources of genetic variants may not be enough to create a comprehensive view of the disease risk. In Kuopio, we are now developing innovative machine learning approaches to combine different sources of data, such as mammographic features", concludes Hamid Behravan, a post-doc researcher specialized in artificial intelligence (AI) and machine learning at Mannermaa cancer research laboratory.
Behravan, H., et al. (2020) Predicting breast cancer risk using interacting genetic and demographic factors and machine learning. Scientific Reports. doi.org/10.1038/s41598-020-66907-9.
"breast" - Google News
July 06, 2020 at 07:39PM
https://ift.tt/2VPXjVC
Using machine learning to analyze the interaction of breast cancer risk factors - News-Medical.Net
"breast" - Google News
https://ift.tt/2ImtPYC
https://ift.tt/2Wle22m
Bagikan Berita Ini
0 Response to "Using machine learning to analyze the interaction of breast cancer risk factors - News-Medical.Net"
Post a Comment